Humanizing Computers
"[AI has the potential to] free humanity from repetitive mental drudgery."
"Life is shockingly short. [With an estimated 27,000 days from birth to death] I don't want to waste that many days."
"It seemed really amazing that you could write a few lines of code and have it [a computer 'neural network'] learn to do interesting things."
"I wish we knew how children [or even a pet dog] learns. None of us today know how to get computers to learn with the speed and flexibility of a child."
Andrew Ng, artificial intelligence specialist, Palo Alto, California
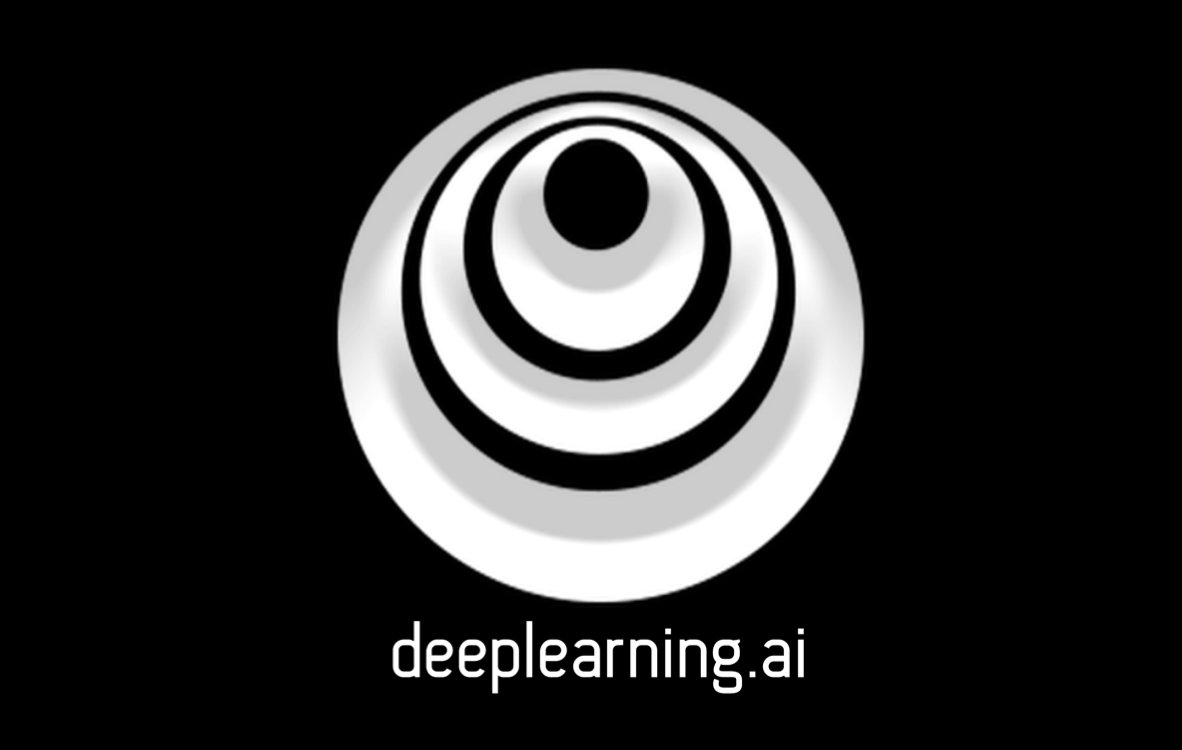
"Several different people suggested using GPUs [to formulate an AI neural network]."The team at University of Toronto led by computer scientist Geoffrey Hinton made use of a neural network to enable them to win the ImageNet competition in 2012, a prestigious AI award. Hinton credits following Andrew Ng's work and identifying it as a platform from which his own research could be usefully based with his success. Credit overall, however, can be given to Mr. Ng for the rise of artificial intelligence as the wave of the future.
"[However, closely following the work by Andrew Ng, the 41-year-old computer scientist] was what convinced me [to use his technique]."
Geoffrey Hinton, computer scientist, University of Toronto
Without leaning on marketing, 100,000 people signed up for Mr. Ng's "Machine Learning" course leading Stanford's online learning program in 2011. The online-learning startup Coursera was co-founded another year on. And now Mr. Ng is preparing to launch deeplearning.ai, producing AI-training courses. Mr. Ng still teaches at Stanford University and at the same time finds the opportunity to work within private industry.
He has led teams that now are capable of creating self-learning computer programs, touching hundreds of millions of people, inclusive of touch-screen keyboards that predict what the users may want to say next. He trained computers to recognize cats in YouTube videos without first informing them what cats were, as a way to lead the machines to learn, unsupervised. He adopted graphics chips meant for video games to artificial intelligence, revolutionizing the field.
Close to two million people globally have taken part in Ng's online course on machine learning to fulfill his focus on teaching the coming generation of AI specialists how to teach machines. He never downplays the difficulties involved in understanding the concepts behind his vision. By the age of six, Ng had learned coding from his father, a medical doctor with a mind to program a computer to use data to diagnose patients.
By age 16 Andrew had written a program to calculate trigonometric functions such as 'sine and cosine' with the use of a 'neural network', the core computing engine of artificial intelligence taking its cue from the human brain. Once he had graduated high school in Singapore, he gained experience at Carnegie Mellon, MIT and Berkeley, finally taking up academic residence as a professor of computer sciences at Stanford University where he taught robotic helicopters aerobatics.
One of Ng's doctoral students, himself now a computer scientist at Berkeley, recalls having once crashed a costly helicopter drone, only to see his supervisor Ng minimize its impact: "Andrew was always like, 'If these things are too simple, everybody else could do them." So Andrew Ng continued to do pioneering AI work that no one else could do; finding a new way to supercharge neural networks with chips used in video-game machines.
Whereas previously computer scientists had relied on general-purpose processors such as Intel chips that operate many PCs, it's recognized that those chips are able to handle a few computing tasks simultaneously at high speed, while neural networks perform more expeditiously if they can run thousands of calculations simultaneously, a task suited to a different chip class, GPUs; graphics processing units.
When Ng's Stanford team began publishing papers on their technique using Nividia's GPUs for general use beyond videos a year later, it was to point out that they had succeeded in speeding up machine learning by up to 70 times with this new technique. Typically, Ng initiates a program, brings it to its conclusion, and when it's up and running leaves it to others he has trained to take it forward.
"Then you go, 'Great. It's thriving with or without me", he says.
![]() |
An example from the new Deep Learning specialization on Coursera -- Coursera webpage |
Labels: Artificial Intelligence, Research
0 Comments:
Post a Comment
<< Home